Guangyao Chen
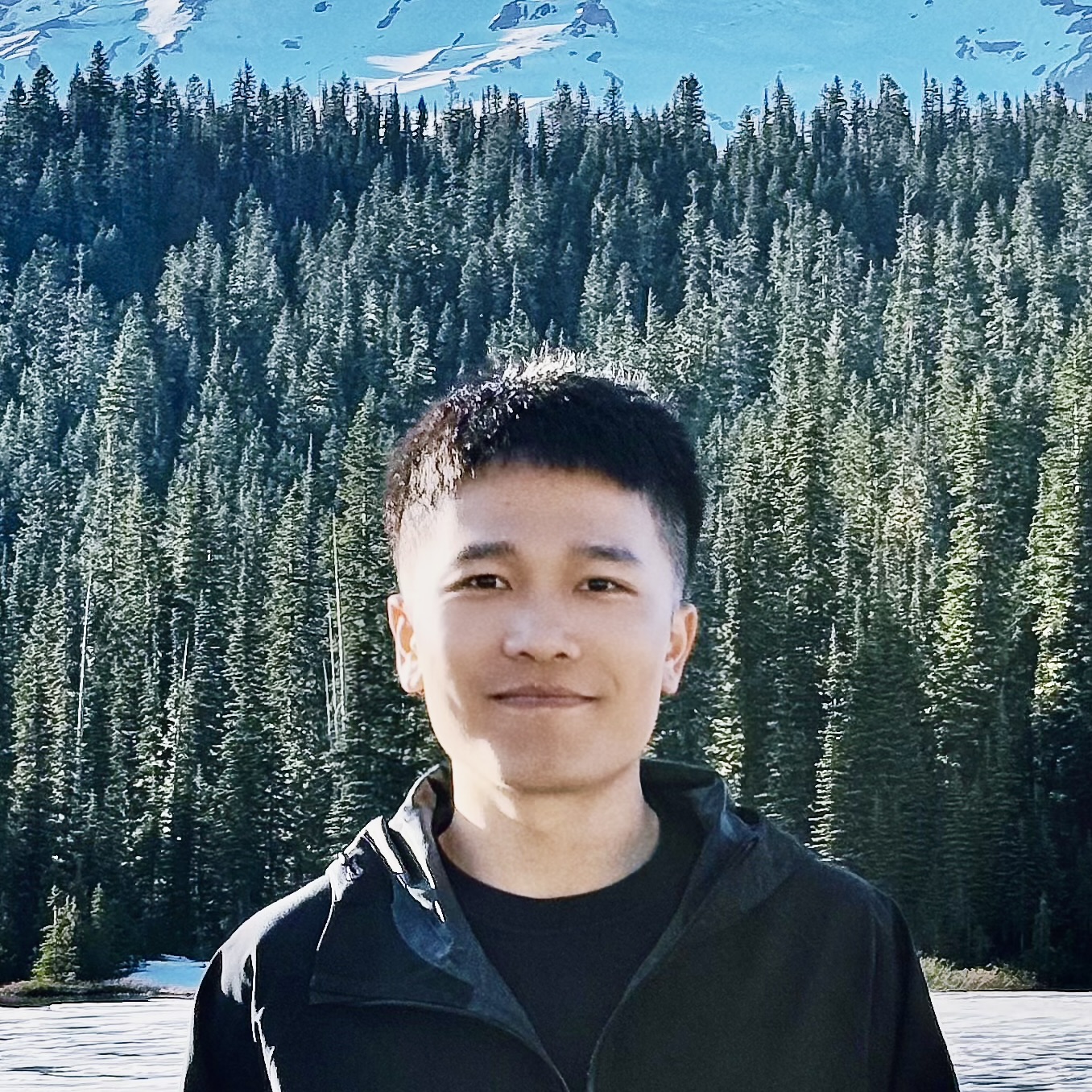
I’m currently a Postdoctoral Researcher at AI for Science Institute, Cornell University. I received my Ph.D. degree at School of Computer Science, Peking University. I received my Bachelor degree in Computer Science from Wuhan University in 2018.
My research vision is to evolve foundation models into lean, reliable, and truly autonomous agents that can learn and act in unrestricted real-world settings. By uniting lifelong adaptation, explainable decision-making, and resource-aware engineering, I aim to create AI systems whose performance and integrity endure as data distributions shift—delivering intelligence that remains robust, trustworthy, and immediately responsive.
- Open-World Learning: continually tune foundation models on diverse, unlabeled data streams so they stay robust to distribution shifts and can recognize novel concepts without forgetting past knowledge.
- Autonomous agents: build lightweight, modular agents that embed planning, memory, and tool use, enabling agent system to collaborate and solve long-horizon tasks autonomously.
- AI for Science: building post-trained science models and automated discovery agents to streamline hypothesis generation, experiments, and analysis across disciplines.
Feel free to catch me if interested to discuss ideas or work together. 😜
news
2025.06 | 🎉🎉 One paper on symbolic reasoning is accepted by the conference ICCV 2025. |
---|---|
2025.05 | 🎉🎉 Three papers (two spotlight papers) are accepted by the conference ICML 2025. |
2025.04 | 🎉🎉 One paper on LLMs for Material is accepted by the journal Advanced Materials. |
2025.03 | 🎉🎉 One workshop paper (spotlight presentation) is accepted by the conference CVPR 2025. |
2024.11 | 🎉🎉 I awared the CSIG 2024 Outstanding PhD Thesis Award (10 people nationwide). |
2024.09 | 🎉🎉 One paper on Visual RL are accepted by the conference NeurIPS 2024. |
2024.07 | 🎉🎉 Two papers on Few-shot Learning are accepted by the conference ACMMM 2024. |
2024.04 | 🎉🎉 One paper on Autonomous Agent is accepted by the conference IJCAI 2024. |
2023.12 | 🎉🎉 One paper on Incremental Novel Class Discovery is accepted by the conference AAAI 2024. |
2023.11 | Invited talk at Qingyuan Workshop (Online). |
2023.11 | Invited talk at RLChina 2023 in Suzhou. |
2023.06 | I receive my Ph.D. degree in computer science from Peking University with Outstanding Doctoral Dissertation Award. |
2022.09 | 🎉🎉 Two papers on Image-based Reinforcement Learning and Out-of-Distribution Detection are accepted by the conference NeurIPS 2022. |
selected publications
-
ICMLWhen Every Millisecond Counts: Real-Time Anomaly Detection via the Multimodal Asynchronous Hybrid NetworkIn Forty-second International Conference on Machine Learning, 2025 (Spotlight Poster, Acceptance Rate: < 2.6%)
-
ACMMMMICM: Rethinking Unsupervised Pretraining for Enhanced Few-shot LearningIn ACM Multimedia, 2024 (Oral Presentation, Acceptance Rate: < 3.97%)
-
ACMMMLearning Unknowns from Unknowns: Diversified Negative Prototypes Generator for Few-shot Open-Set RecognitionIn ACM Multimedia, 2024